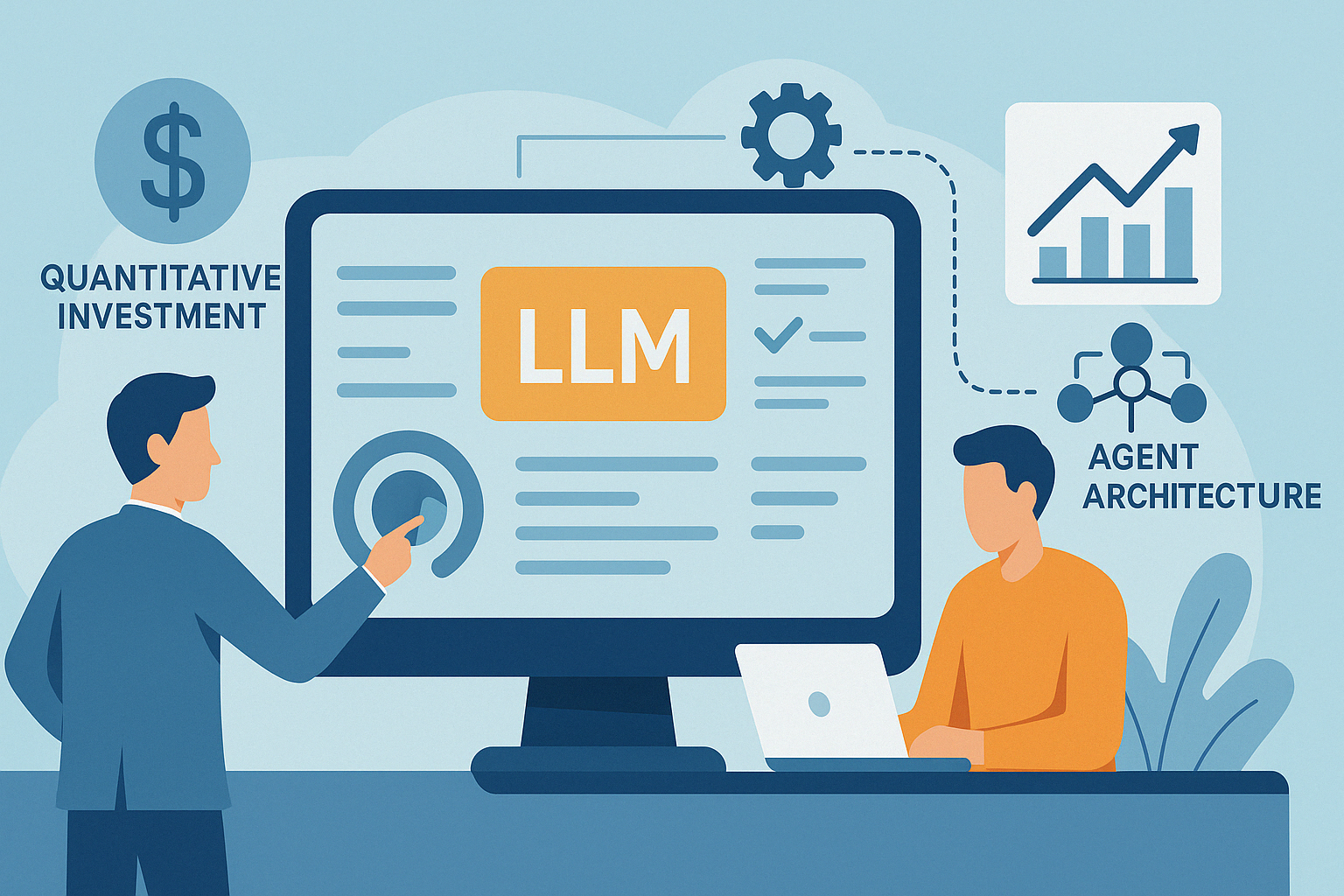
Agents in Formation: Fine-Tune Meets Fine-Structure in Quant AI
The next generation of quantitative investment agents must be more than data-driven—they must be logic-aware and structurally adaptive. Two recently published research efforts provide important insights into how reasoning patterns and evolving workflows can be integrated to create intelligent, verticalized financial agents. Kimina-Prover explores how reinforcement learning can embed formal reasoning capabilities within a language model for theorem proving. Learning to Be a Doctor shows how workflows can evolve dynamically based on diagnostic feedback, creating adaptable multi-agent frameworks. While each stems from distinct domains—formal logic and medical diagnostics—their approaches are deeply relevant to two classic quant strategies: the Black-Litterman portfolio optimizer and a sentiment/technical-driven Bitcoin perpetual futures trader. ...