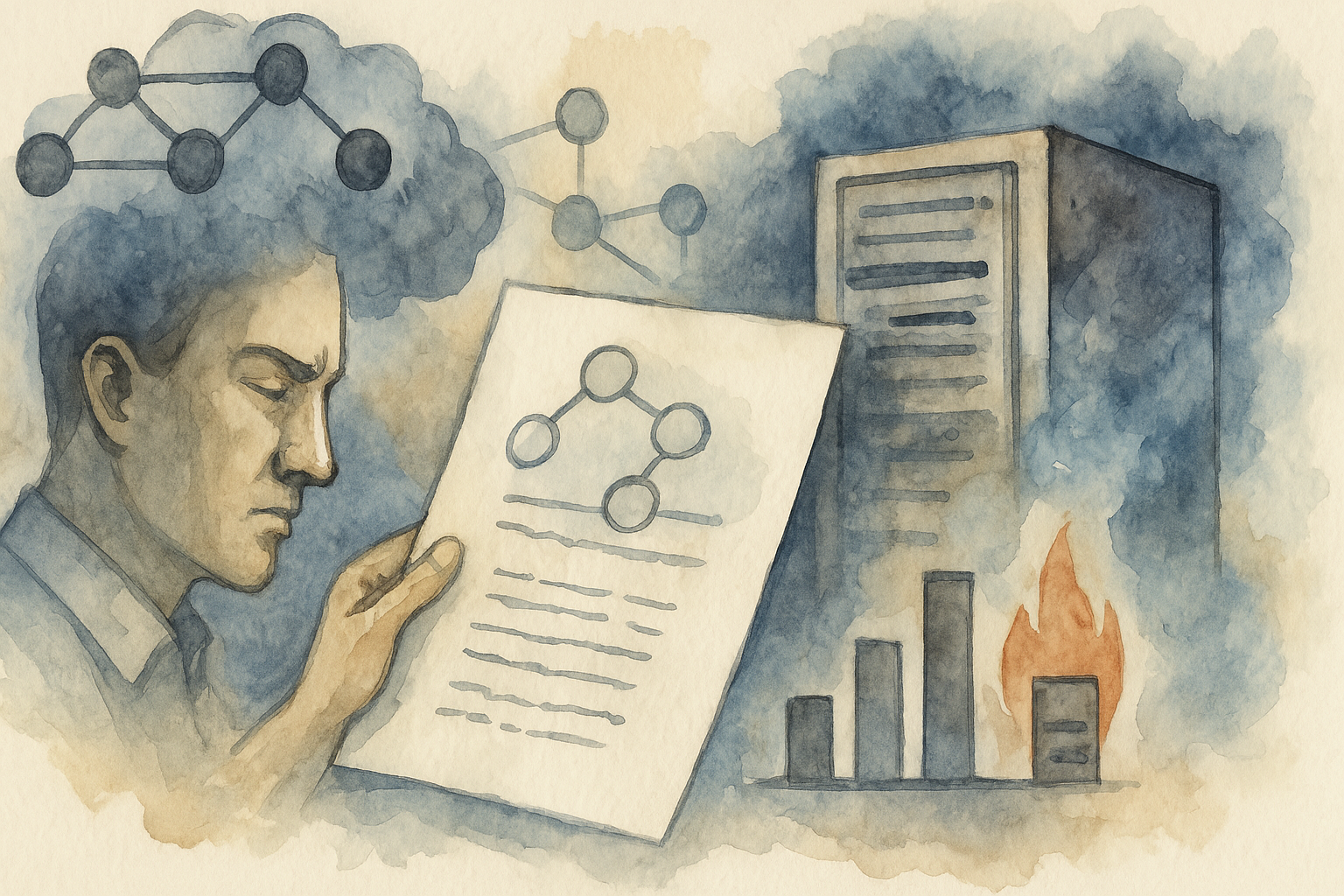
Cut the Fluff: Leaner AI Thinking
Cut the Fluff: Leaner AI Thinking When it comes to large language models (LLMs), brains aren’t the only thing growing—so are their waistlines. As AI systems become increasingly powerful in their ability to reason, a hidden cost emerges: token bloat, high latency, and ballooning energy consumption. One of the most well-known methods for boosting LLM intelligence is Chain-of-Thought (CoT) reasoning. CoT enables models to break down complex problems into a step-by-step sequence—much like how humans tackle math problems by writing out intermediate steps. This structured thinking approach, famously adopted by models like OpenAI’s o1 and DeepSeek-R1 (source), has proven to dramatically increase both performance and transparency. ...