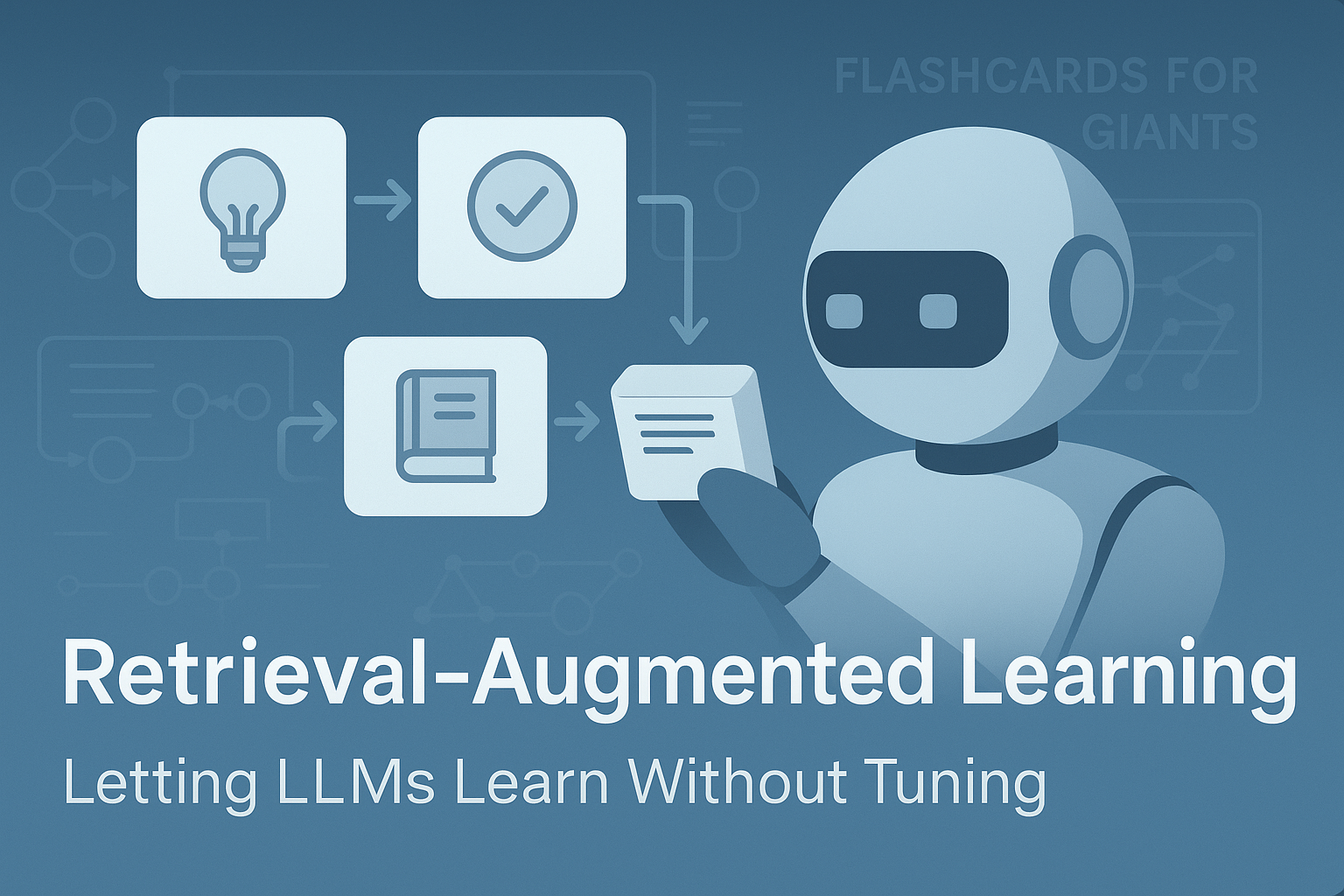
Flashcards for Giants: How RAL Lets Large Models Learn Without Fine-Tuning
Cognaptus Insights introduces Retrieval-Augmented Learning (RAL), a new approach proposed by Zongyuan Li et al.¹, allowing large language models (LLMs) to autonomously enhance their decision-making capabilities without adjusting model parameters through gradient updates or fine-tuning. Understanding Retrieval-Augmented Learning (RAL) RAL is designed for situations where fine-tuning large models like GPT-3.5 or GPT-4 is impractical. It leverages structured memory and dynamic prompt engineering, enabling models to autonomously refine their responses based on previous interactions and validations. ...