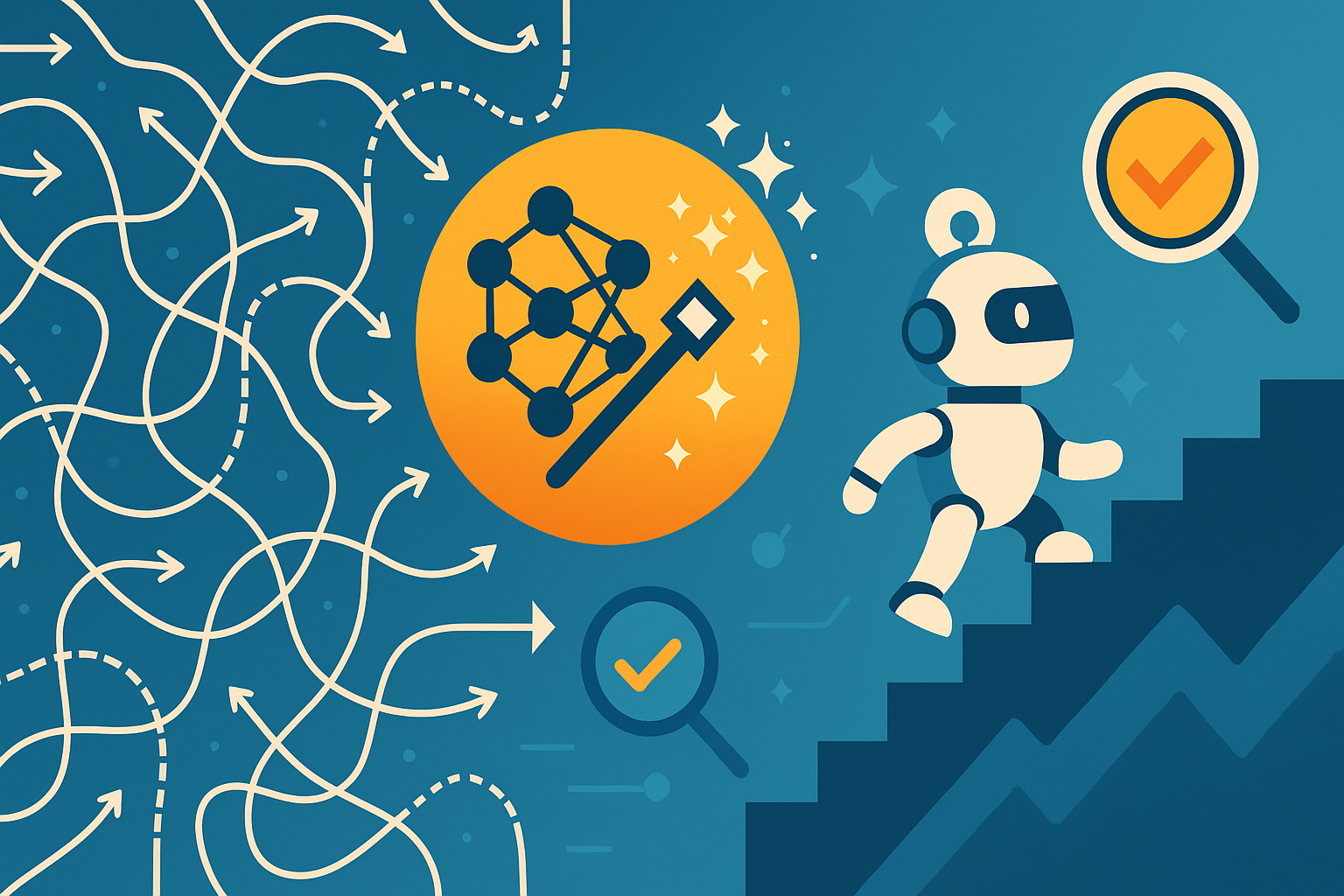
From Infinite Paths to Intelligent Steps: How AI Learns What Matters
Training AI agents to navigate complex environments has always faced a fundamental bottleneck: the overwhelming number of possible actions. Traditional reinforcement learning (RL) techniques often suffer from inefficient exploration, especially in sparse-reward or high-dimensional settings. Recent research offers a promising breakthrough. By leveraging Vision-Language Models (VLMs) and structured generation pipelines, agents can now automatically discover affordances—context-specific action possibilities—without exhaustive trial-and-error. This new paradigm enables AI to focus only on relevant actions, dramatically improving sample efficiency and learning speed. ...